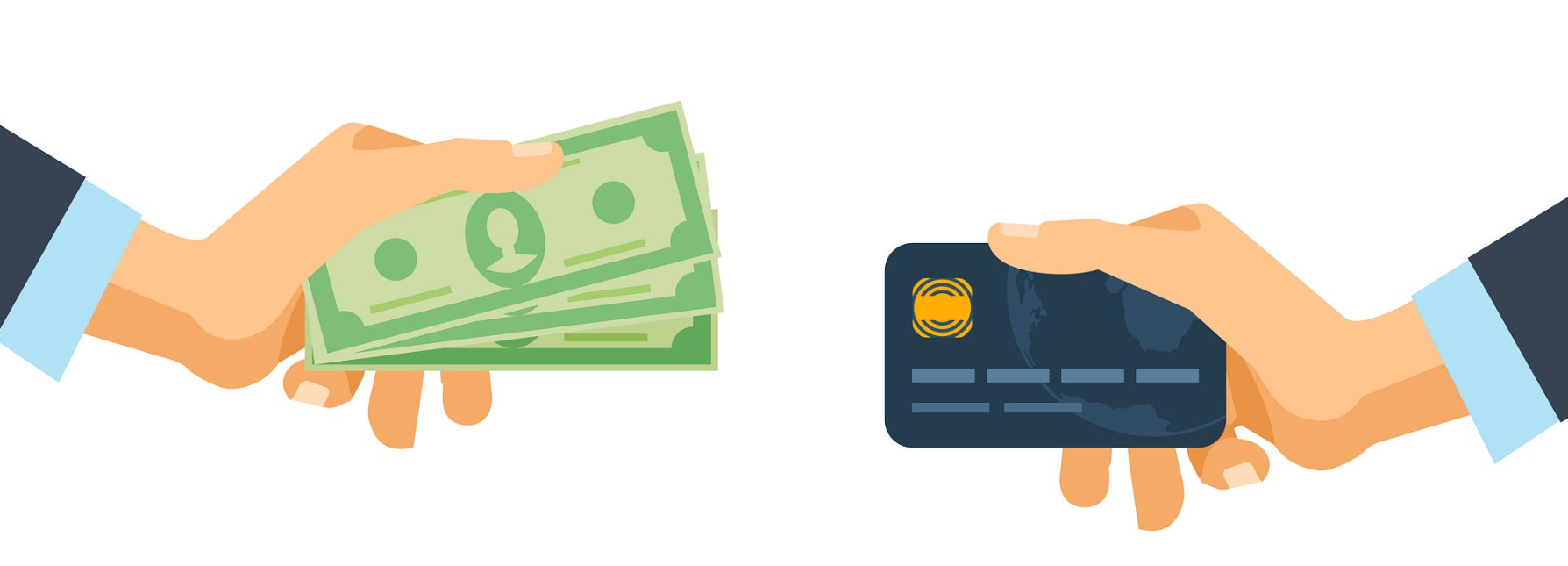
Predict which credit card customers will default on their credit card loans to strengthen credit card portfolio.
Default poses a persistent challenge within the credit card industry, presenting considerable hurdles for lenders to navigate effectively. The limited capacity to comprehensively analyze information surrounding credit card applicants exacerbates the difficulty in identifying all potential credit risks associated with borrowers. According to insights from Wells Fargo, a leading financial institution, the largest U.S. banks derive a substantial portion of their revenue, approximately 15%, from their credit card portfolios. However, the inaccurate judgment of applicants' creditworthiness results in significant revenue losses amounting to billions of dollars annually for banks across the sector.
In light of these challenges, a core growth strategy for banks revolves around expanding the pool of consumers who are offered credit. This strategy is essential for driving revenue growth and expanding market share. However, the primary challenge lies in effectively assessing the risk of entering into relationships with new credit card consumers. The inherent complexity of this task underscores the critical need for advanced analytical tools and methodologies to enhance the accuracy and efficiency of credit risk assessment processes.
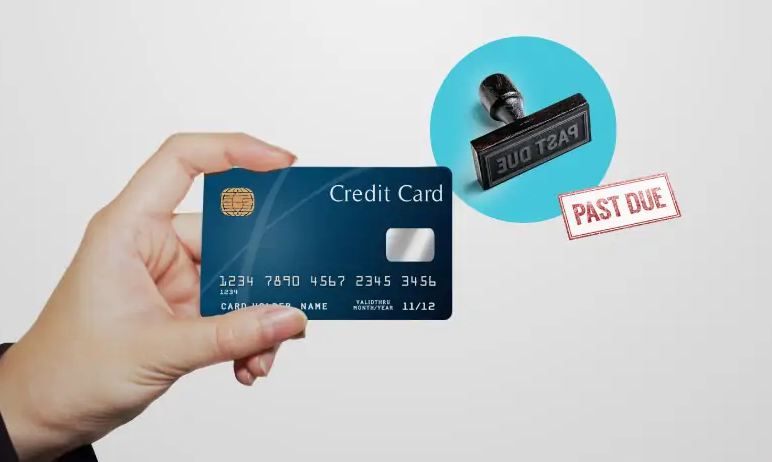
By leveraging innovative technologies such as artificial intelligence and machine learning, banks can enhance their ability to analyze vast volumes of data and identify patterns indicative of potential credit risks. These advanced analytical capabilities enable lenders to make more informed decisions when evaluating credit card applicants, thereby reducing the likelihood of default and minimizing revenue losses associated with inaccurate risk assessments.
Furthermore, a proactive approach to credit risk management involves implementing robust risk mitigation strategies and continuously monitoring the credit performance of existing cardholders. By identifying early warning signs of potential default and taking proactive measures to mitigate risks, banks can minimize their exposure to credit losses and maintain the overall health of their credit card portfolios.
In summary, while default remains a persistent challenge within the credit card industry, banks have the opportunity to mitigate risks and drive revenue growth by adopting advanced analytical tools and implementing proactive risk management strategies. By enhancing their ability to assess credit risk accurately and efficiently, banks can minimize revenue losses associated with default and position themselves for sustainable growth in an increasingly competitive market landscape.
Artificial Intelligence (AI) emerges as a scalable and sophisticated solution tailored for credit card lenders grappling with the challenge of assessing default risk. By harnessing AI-driven predictive modeling techniques developed on the foundation of historical default data, lenders gain a powerful tool to evaluate the likelihood of credit card defaults with precision and efficiency.
Beyond the conventional reliance on consumer credit scores, AI models introduce a comprehensive approach by incorporating a diverse array of consumer data points. This expanded dataset enables AI algorithms to delve deeper into the intricate nuances of individual borrower profiles, thereby enhancing the accuracy of predictions regarding the propensity of a credit card to transition into default status.
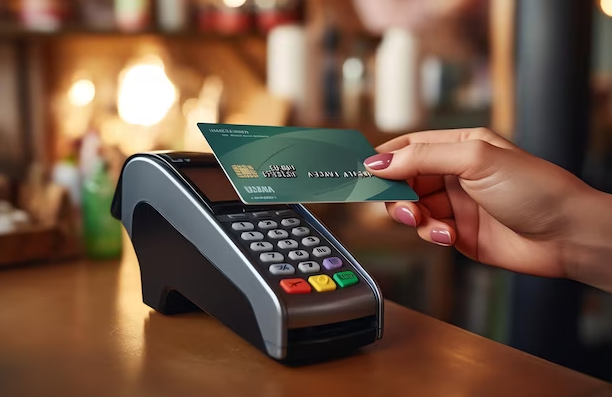
Integrating these predictive insights into the application decision-making process empowers organizations to discern and stratify applicants based on their creditworthiness and likelihood of fulfilling their credit card debt obligations. By leveraging AI-driven risk assessment tools, lenders can effectively identify and prioritize applicants deemed less susceptible to default, thereby optimizing portfolio management and minimizing potential credit losses.
Moreover, the evolution of AI-driven machine learning techniques has facilitated significant advancements in model interpretability. This enhancement enables lenders to gain deeper insights into the underlying statistical factors driving the classification of each credit card as either high or low risk. By understanding the rationale behind these risk classifications, organizations can refine their decision-making processes and implement targeted risk mitigation strategies tailored to specific borrower profiles.
By embracing AI-driven credit risk assessment solutions, organizations can not only mitigate the financial impact associated with charge-offs but also streamline operational processes related to debt collection and risk management. This proactive approach not only safeguards the organization's bottom line but also fosters operational resilience and agility in navigating dynamic market conditions.
In essence, the adoption of AI-powered credit risk assessment tools represents a strategic imperative for credit card lenders seeking to enhance portfolio performance, optimize risk management practices, and foster sustainable growth in an increasingly competitive landscape. Through the integration of advanced analytics and machine learning capabilities, organizations can unlock new opportunities to drive operational efficiency, mitigate credit losses, and deliver enhanced value to stakeholders.
Copyright © 2023 Wizio AI